3.1 Overview of suggested approach
Chapter 3: Suggested Educational ApproachAn overview of the suggested approach.
The insights provided in the first report1 show the importance of broad educational efforts across the NHS workforce. Interviewees for this research observed that in many health settings, industry innovators are currently shaping the workforce’s knowledge of artificial intelligence (AI), which highlights the importance of vendor-neutral educational materials, and sources that highlight the potential value, limitations and risks of AI for healthcare. Ultimately, healthcare workers should be supported to critically appraise the technologies, evidence and information provided by private sector providers.
As noted by interviewees, the broad educational challenge is to provide the right resources to the right people, and to build skills and capabilities across the workforce in the most efficient and effective way possible.
This approach to educating the healthcare workforce can be informed by previous experiences within the NHS learning, both in terms of what has worked well and what hasn’t. Examples include the introduction of computers, the introduction of imaging management software in radiology and the transition to electronic health records (EHRs). These transitions required a mixture of widespread training and skills acquisition, whilst also establishing specialist technical teams with the expertise to deploy and support these technologies.
Similarly, basic understanding of AI will be required for all healthcare workers who might use such technologies, while further knowledge and skills requirements will depend on profession, roles and responsibilities related to AI, the technologies being used, and the settings where they are deployed.
Based on these observations, Figure 3 illustrates the suggested approach to education and training for AI in healthcare.
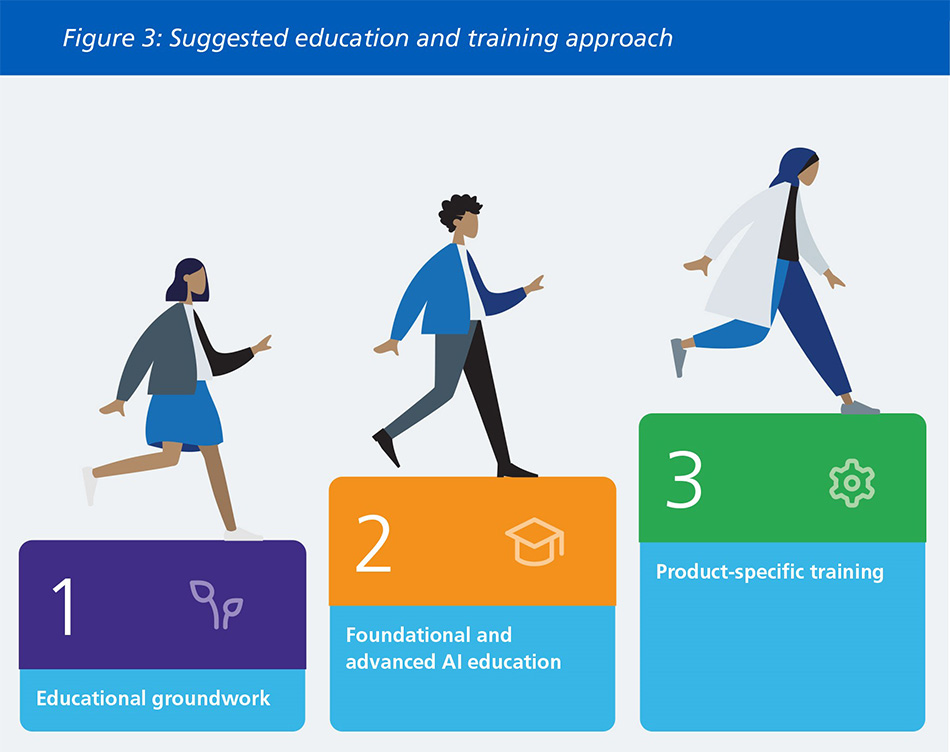
The suggested education and training approach outlined in Figure 3 is as below.
- An essential groundwork to AI education and training, continuation of the broader efforts to enable the adoption of change and innovation in healthcare settings, as well as efforts to advance digital literacy, skills, and capabilities within the workforce (such as the digital transformation pathways outlined in the NHS Long Term Plan, the Topol and Goldacre reviews, the plan for digital health and social care, and ‘Data saves lives’ strategy).
- Development and provision of general education and training for AI through a) foundational AI education across the whole healthcare workforce, including basic AI literacy and awareness of the limitations and risks of using AI technologies b) advanced AI education that is specific to the workforce archetypes, including development of skills and capabilities.
- Provision of product-specific training for users of each AI technology during its deployment.
Knowledge, skills and capabilities taxonomies
The requirements for knowledge, skills and capabilities in this chapter and Appendix A are organised according to progressive taxonomies. The taxonomies, illustrated in Figure 4, have been developed as a pragmatic approach to define levels of required knowledge and skills, with implications for the nature and degree of related education and training efforts.
The taxonomies have been informed by other existing approaches to education and training, including Bloom’s taxonomy19 and competence-based approaches to skills hierarchies.20
The taxonomies are based on the principle that knowledge underpins skills, which requires theoretical understanding to underpin practical application. Skills and capabilities enable people to perform a task, contribute to, or lead an aspect of a project.
Figure 4: Taxonomies
The following statements summarise the progressive outcome of knowledge-based education and training efforts from a learner’s perspective.
- Awareness: ‘I am aware of this concept and its relevance to my healthcare practice’.
- Familiarity: ‘I know more detail about this topic and can use this knowledge to guide my practice and make decisions’.
- Understanding: ‘I understand this subject in depth and can synthesise my knowledge to apply it to new and challenging situations. I can teach and support others within my area of practice’.
The following statements summarise the progressive outcome of skills-based education and training efforts from a learner’s perspective.
- Capability: ‘I am able to perform this task either independently or as part of a team’.
- Proficiency: ‘I am experienced in this area and can lead on this aspect of a project, guiding and educating others to drive success’.
The following statements summarise the progressive outcome of knowledge-based education and training efforts from a learner’s perspective.
- Awareness: ‘I am aware of this concept and its relevance to my healthcare practice’.
- Familiarity: ‘I know more detail about this topic and can use this knowledge to guide my practice and make decisions’.
- Understanding: ‘I understand this subject in depth and can synthesise my knowledge to apply it to new and challenging situations. I can teach and support others within my area of practice’.
The following statements summarise the progressive outcome of skills-based education and training efforts from a learner’s perspective.
- Capability: ‘I am able to perform this task either independently or as part of a team’.
- Proficiency: ‘I am experienced in this area and can lead on this aspect of a project, guiding and educating others to drive success’.
Progression from awareness to understanding suggests increasing exposure to educational materials that can enable learners to synthesise and critique information, in the spirit of Bloom’s educational taxonomy. If an individual ‘understands’ a given topic they can be assumed to also have awareness and familiarity with that topic.
Capability implies a level of experience appropriate for contributing to a project as part of a wider team. Proficiency is associated with greater experience and expertise and implies the ability to lead an aspect of the project. If an individual is ‘proficient’ in a skill, they can be assumed to be capable and to have appropriate understanding of the subject.
For both knowledge and skills, the educational content required will vary with each taxonomy level. The details of the provided education, and how it is assessed, will depend on the professional group, career level and role of the individual. These factors need to be considered when developing curricula informed by this approach.
References
1 Nix M, Onisiforou G, Painter A. Understanding healthcare workers’ confidence in AI. Health Education England & NHS AI Lab. 2022. https://digital-transformation.hee.nhs.uk/binaries/content/assets/digital-transformation/dart-ed/understandingconfidenceinai-may22.pdf Accessed 29 June, 2022.
19 Bloom B. Taxonomy of Educational Objectives, Handbook I. 1956. Addison Wesley Publishing Company.
20 Bowers D, Petre M, Howson O. Aligning Competence Hierarchies with Bloom's Taxonomies: Changing the focus for computing education. In: Proc. of 19th Koli Calling International Conference on Computing Education Research. 2019. ACM.
Page last reviewed: 18 April 2023
Next review due: 18 April 2024